A Pandemic Toolkitgoing beyond contact tracing
Our COVID-19 research examines how technology can help respond to pandemics, e.g., by developing techniques for real time contact tracing and risk monitoring via privacy-enhanced mobility tracking. We have integrated our research results in a Pandemic Toolkit. You will find below details on the research.
Privacy-enhanced contact tracing and risk monitoring
We examine Rigorous and customizable privacy-enhancing mechanisms for mobility data collection and analysis to enable privacy-enhanced contact tracing and risk monitoring and understand their privacy/utility trade off. In contact tracing human operators interview cases one at the time and follow-up with potential contacts. To scale this model requires the number of contact tracers to be proportional to the number of cases and therefore lacks scalability. In addition, it relies on recollection which is often not sufficient to identify contacts potentially exposed. Digital contact tracing has the potential to alleviate these challenges. Many contact tracing applications use Bluetooth-based proximity only, not absolute locations, to protect privacy. However, ignoring absolute locations sacrifices the ability to estimate the fine-grained transmission risk based on the type of the locations and identified hot spots. We have been designing and developing algorithms and mobile app that use location information (in addition to proximity information) for more effective contact tracing while at the same time ensure location privacy. The key is to develop efficient and scalable spatiotemporal data structures and algorithms given the potentially large number of users and location traces. A critical issue in using real-time location traces of users for digital contact tracing is user privacy. We have been investigating existing location privacy solutions such as location perturbation and their use for contact tracing and monitoring. The main challenge is to accurately compute the contact (range or reachability queries) or the risks based on the perturbed locations. In addition, we observed that existing one-size-fits-all approach may not fit every application’s requirement on utility-privacy trade-off. Different tasks, e.g. individual based contact tracing vs. aggregate risk monitoring, may have different usage of the data and thus need different location privacy policies to strike the right balance between privacy and utility. We have been investigating rigorous and customizable privacy notions based on privacy policies
We developed algorithms and a mobile app (forked from an existing open source project named Covid Community Alert) for contact tracing using mobility data. It uses both proximal contacts (via Bluetooth) and locations (via GPS if permissed by user). Using the absolute (privacy-enhanced) locations by GPS can alleviate or address many of the shortcomings of existing approaches (e.g., Google and Apple Bluetooth Apps) that use only proximity information.
Developing Fine-Grained Risk Scores
While contact tracing only aims to track past activities of infected users, one path to controlling the spread of COVID-19 is to develop reliable spatiotemporal risk scores to indicate the risk of contracting the disease in a particular area and for a specific period of time. Existing works which consider this problem develop coarse-grain spatial scores, e.g., at a city level, which provides no useful information for both individual and policy-making plans. Such coarse-grain risk scores are not meaningful, as the risk of contracting a disease varies vastly across the city, and depends on the specific area (e.g., risk of infection in a mall vs. at home). We have studied the problem of developing fine-grained spatiotemporal risk scores.
Simulating disease spread: SpreadSim
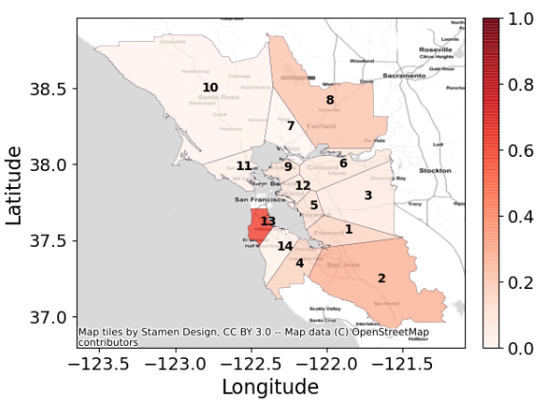
We developed a disease spread simulator using real mobility data. As a result of our collaboration with Microsoft Research and Veraset, a data-as-a-service company, we were provided with fine-grain real-world cellphone originated location signals of individuals across the US. We used such mobility information, as well as information known about COVID-19, e.g., the incubation period and recovery time to build a simulator, named SpreadSim.
COVID Community Alert App
We have developed a concept application to collect data using the community App (e.g., for USC) for individuals (e.g., USC students) to voluntarily contribute data. The App can record symptoms (momentary assessments) with location from scanned barcodes placed at the entrance of buildings or rooms, and producing location diaries. The addition of privacy techniques allows preserving the privacy of the users. This allows to collect more data (e.g., locations, traces, durations), plus co-locations that can be used in a contact tracing application and allows for less false positives and can detect fomites and indirect transmissions. App users are provided with a risk score based on their whereabouts, and not alerts as is the case in existing Apps, which is more consistent with how we use Apps.
Contact Tracing Dashboard
We have developed a prototype contact tracing dashboard that allows contact-tracing by professionals with the help of geospatial and contact data, i.e., to onboard confirmed cases, perform spatial and temporal search of exposed, risk prioritization of potential exposed for processing and confirmed follow-up. The contact tracing dashboard addresses problems with memory recall, scale and latency and supports privacy-preserving computation and other usage of the collected data such as location risk scores and inform spread models.
CrowdMap
In addition to using mobility data directly collected from users’ mobile phones, we have used wifi data as an alternative data source for monitoring occupancy and risks. Occupancy information in an institutional setting or workplace is essential to help ensure compliance with distancing policies and support contact tracing. Several technology solutions have been proposed to help collect this information with various levels of privacy. Collecting occupancy information consistently for a large institution such as a university campus in a private manner is challenging. We designed an end-to-end system that demonstrates how occupancy information can be collected anonymously using WiFi access points for the entire University of Southern California (USC) and created a dashboard dubbed CrowdMap where this information is presented to help monitor and ensure compliance with social distancing policies. CrowdMap estimates the number of people at a location based on the number of devices connected to the corresponding WiFi access points (AP), thereby providing 24/7 anonymous occupancy monitoring for all access point locations on campus. With CrowdMap we leverage these anonymized AP logs to compute occupancy at four levels: AP, floors, buildings, and campus. In spaces with large capacity (e.g., labs and classrooms) multiple APs are used to optimize WiFi signal to users, which provides a fine-grained spatial correlation between people and workspaces. The CrowdMap dashboard provides authorized users with occupancy analytics data since July 2020.
Researchers: Cyrus Shahabi (USC), Gabriel Ghinita (UMass Boston), Li Xiong (Emory University), Vicki Hertzberg (Emory University), Lance Waller (Emory University), John Krumm (Microsoft), Xiaoqian Jiang (University of Texas) Amy Franklin (University of Texas), Luciano Nocera (USC) PHD students: Ritesh Ahuja, Sitao Min, Kien Nguyen, Sina Shaham, Sepanta Zeighami Undergraduate students: Yingzhe Liu, Catherine Phu, Kameron Shahabi, Abbas Zaidi
Sponsors
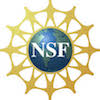
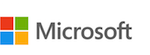
External links
- NSF REACT Project website
- Why We Need More Than Bluetooth Data to Fight Covid-19, Cyrus Shahabi, Medium article, Apr. 2020.
- Digital contact tracing goes beyond mobile phones, reducing false positives, Cyrus Shahabi, Viterbi News, Nov. 2020